一種新的基于數(shù)據(jù)挖掘技術的異常入侵檢測系統(tǒng)研
輸入:數(shù)據(jù)信息E,滑動窗口T,時間t,相似度m,窗口個數(shù)k,步長t0,數(shù)據(jù)挖掘規(guī)則庫K,待測數(shù)據(jù)規(guī)則為V。
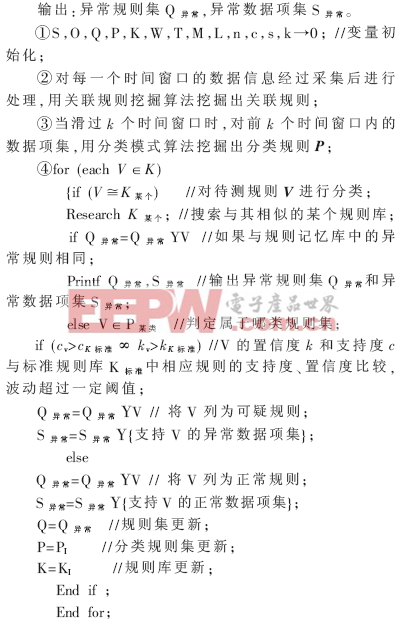
⑤if W={異常}重復②、③、④ //對滑動時間窗口得到數(shù)據(jù)規(guī)則集進行數(shù)據(jù)評估;
L=W //每次檢測結果提交決策列表以供用戶決策;
5 實驗分析
數(shù)據(jù)參考MIT林肯實驗的DARPA 1999年評測數(shù)據(jù)集。由于數(shù)據(jù)信息自身的復雜性,需要對數(shù)據(jù)信息進行多次訓練以降低數(shù)據(jù)噪音的影響。在本實驗中對ANEIDSDM算法進行模擬測試分為兩個階段:
(1)為數(shù)據(jù)訓練階段:首先收集數(shù)據(jù)信息,依此數(shù)據(jù)信息對其抽取特征主屬性,挖掘高頻度數(shù)據(jù)項集和低頻數(shù)據(jù)項集,對高頻數(shù)據(jù)項集進行數(shù)據(jù)模式集,對數(shù)據(jù)模式集進行數(shù)據(jù)挖掘,形成數(shù)據(jù)規(guī)則集,最后對數(shù)據(jù)規(guī)則集進行分類,形成標準規(guī)則庫。實驗時分為3個階段收集,實現(xiàn)3次訓練,如表1所示。
(2)數(shù)據(jù)模擬檢測階段:對待測數(shù)據(jù)信息進行數(shù)據(jù)規(guī)則集的挖掘,根據(jù)與標準規(guī)則庫中分類規(guī)則集的相似度對比,快速分類,通過在線滑動窗口和匹配檢測方法,對數(shù)據(jù)信息進行異常入侵檢測。若屬于異常信息,則進行預警。實驗時通過對7種常見攻擊類型的模式進行異常入侵檢測,如表2所示。
通過模擬攻擊實驗表明,數(shù)據(jù)信息經(jīng)過ANEIDSDM入侵檢測能夠很好地檢測異常數(shù)據(jù)信息,其誤警率和檢測率都有了明顯的提高。本實驗同時可以有效地提高入侵檢測系統(tǒng)的檢測速度。
本文針對現(xiàn)有異常入侵檢測系統(tǒng)存在的問題,建立了一種新的基于數(shù)據(jù)挖掘技術的異常入侵檢測系統(tǒng)模型。該模型包括數(shù)據(jù)采集、數(shù)據(jù)分析、數(shù)據(jù)評估、事件響應等一系列檢測過程,利用多次訓練、滑動窗口、規(guī)則分類和相似度匹配思想,大大降低了系統(tǒng)的誤警率,提高了檢測速度,提升了檢測率,增強了網(wǎng)絡系統(tǒng)的安全性能。
參考文獻
[1] VERWORD T,HUNT R. Intrusion detection techniques and approaches[J].Computer Communication,2002,25(15): 1356.1365.
[2] LANE T. Machine learning techniques for the computer security domain of anomaly detection[D]. Purdue University,2000.
[3] MUKKAMALA S, SUNG A H,ABRAHAM A. Intrusion detection using all ensemble of intelligent paradigms[J].Journal of Network and Computer Application,2005,28(2):167-182.
[4] 呂志軍,袁衛(wèi)忠,仲海駿,等. 基于數(shù)據(jù)挖掘的異常入侵檢測系統(tǒng)研究[J].計算機科學,2004,31(10):61-65.
[5] 田新廣,李文法,段σ,等. 基于數(shù)據(jù)挖掘和變長序列模式匹配的程序行為異常檢測[J].信號處理,2008,24(4):521-555.
[6] 凌軍,曹陽,尹建華,等. 基于時態(tài)知識模型的網(wǎng)絡入侵檢測方法研究[J].計算機學報,2003,26(11):1591-1597.
[7] 楊向榮,宋擒豹,沈鈞毅,等. 基于數(shù)據(jù)挖掘的智能化入侵檢測系統(tǒng)[J].計算機工程,2001,27(9):17-102.
[8] BARFORD P,HIINE J,PLONKA D,et al. A signal analysis of network traffic anomalies[J].Internet Measurement Workshop,2002,7:1-82.
[9] YE N, LI Xiang Yatig,CHEN Qiang. Probabilistic techniques for intrusion detection based on computer audit data[J]. Man and Cybernetics,Part A,IEEE Transactions on 2001:31(4):266-274.
[10] YE N,EMRAN S M,CHEN Q, et a1. Multivariate statistical analysis of audit trails for host-based intrusion detection[J].IEEE Transactions on Computers,2002,51(7):810-820.
[11] OH S H,LEE W. A clustering based anomaly intrusion detection for a host computer[J].IEICE Transactions on In.formation and Systems,2004,E87-D(8):2086-2094.
[12] HOFMEYR S A,F(xiàn)ORREST S,SOMAYAJI A. Intrusion detection using sequences of system calls[J]. Journal of Computer Security,1998(6):151-180.
[13] LANE T,CARLA E B. An empirical study of two approaches to sequence learning for anomaly detection[J].Machine Learning,2003,51(1):73-107.
評論